In a new perspective piece “Transparency and reproducibility in artificial intelligence” published this week in the journal Nature, an international group of scientists including CUNY Graduate School of Public Health and Health Policy (CUNY SPH) Associate Professor and ISPH investigator Levi Waldron raised concerns about the lack of transparency in publication of artificial intelligence algorithms for health applications.
The authors raise concerns about a recent publication in which a group including Google Health reported using artificial intelligence to diagnose breast cancer from mammogram images more accurately than expert human radiologists. The authors contend that restrictive data access procedures, lack of published computer code, and unreported model parameters make it impractically difficult for any other researchers to confirm or extend this work. The piece also highlights tensions over what are appropriate measures to protect patient privacy while allowing the broader research community to contribute methodology and to correct potential errors that could set back progress to the detriment of other patients.
“This back-and-forth is one high-profile example of the current state of struggles over who controls data that has played out for decades in the biomedical sciences and other fields,” says Professor Waldron. “Well-funded researchers who collect patient data have little incentive to share, but they are the ones who write the informed consent asked of patients and who dictate the terms of sharing. Protecting patient privacy from even the most hypothetical of risks can become a method to keep invaluable data, and even the parameters of resulting prediction models, away from other researchers. I don’t think the patients volunteering for medical research are informed of the trade-offs between data privacy and utility, or are given much say in them. This is a bigger conversation that should happen above the level of individual research studies, and it needs to include patients.”
About the Institute for Implementation Science in Population Health at the City University of New York
The CUNY Institute for Implementation Science in Population Health (ISPH) was founded on the notion that substantial improvements in population health can be efficiently achieved through better implementation of existing strategies, policies, and interventions across multiple sectors. We study how to translate and scale-up evidence-based interventions and policies within clinical and community settings in order to improve population health and reduce health disparities. CUNY ISPH. Pursuing population health gains through better implementation. www.cunyisph.org. Follow us on Twitter: @CUNYISPH.
About the CUNY Graduate School of Public Health and Health Policy
The CUNY Graduate School of Public Health and Health Policy (CUNY SPH) is committed to teaching, research and service that creates a healthier New York City and helps promote equitable, efficient and evidence-based solutions to pressing health problems facing cities around the world. For more information, visit sph.cuny.edu.
References
[1] McKinney, S. M. et al. International evaluation of an AI system for breast cancer screening. Nature 577, 89–94 (Jan 1, 2020)
[2] Haibe-Kains, B. et al. Transparency and reproducibility in artificial intelligence. Nature (Oct 14, 2020)
[3] McKinney, S.M. et al. Reply to: The importance of transparency and reproducibility in artificial intelligence research. Nature (Oct 14, 2020)
[4]Grady D. “A.I. Is Learning to Read Mammograms.” The New York Times, (Jan 1, 2020)
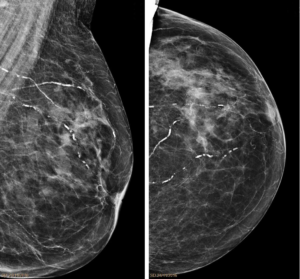